2021 has been an exciting year for our researches working on the recognition of emotions from voice. Benefiting from the recent advances in transformer-based architectures, we have for the first time built models that predict valence (i.e. how negative or positive an utterance sounds) with a similar high precision as arousal (i.e. how excited an utterance sounds). Closing the valence gap has been a major challenge in speech emotion recognition (SER). And unlike previous approaches, our models derive their predictions directly from the raw audio and do not require text transcriptions.
Valence prediction without use of linguistic information
The sparseness of labeled data is one of the biggest barriers in SER research. To overcome this limitation we have now shifted our focus from specialised architectures trained for a given task towards general-purpose foundation models. Foundation models are trained in an unsupervised manner on large datasets without labels and afterwards fine-tuned on (small) sets of labelled data for their intended tasks. In a joint publication between audEERING and the Chair for Embedded Intelligence for Health Care and Wellbeing (University of Augsburg) under the supervision of CSO Prof. Björn Schuller, we provide a thorough evaluation of this approach with respect to generalisation, robustness, fairness, and efficiency. Our proposed system obtains top performance for valence prediction without use of explicit linguistic information, with a concordance correlation coefficient (CCC) of 0.638 on MSP-Podcast.

Tutorial available for further analysis
To encourage the community to benchmark against our approach and do further analysis, we have made the best performing model from the paper publicly available. A tutorial shows how to use the model and highlights another strength: the output of intermediate layers (so called embeddings) provide a compact speech representation that generalizes well to other emotion tasks. We show that the learned representations can even outperform classical hand-crafted features like the popular ComParE 2016 set from openSMILE.
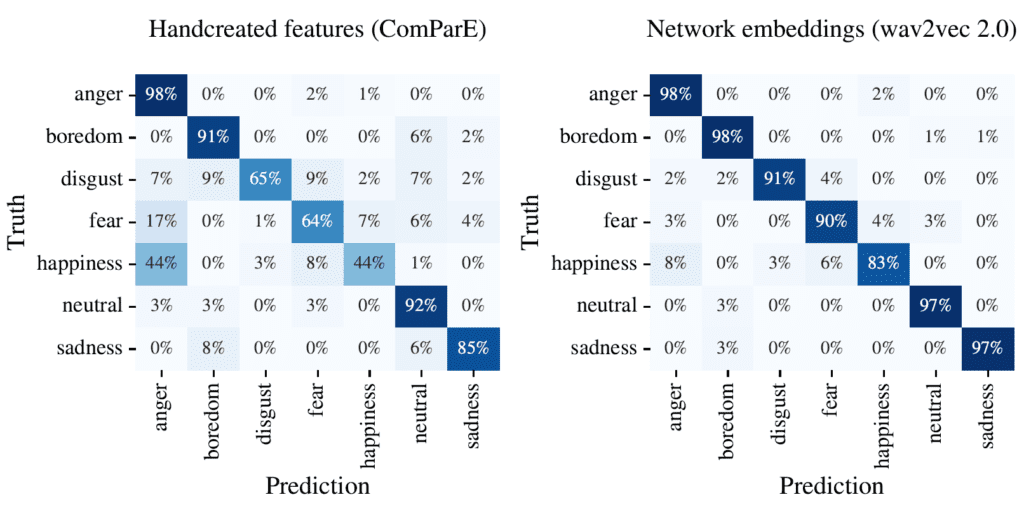
Two steps ahead
With the latest update the new technology is already available in our product devAIce.