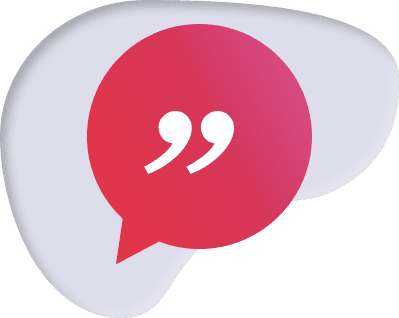
Citations
audEERING’s technology is used in numerous research projects. Below you will find a selection of references and citations from various fields. Please also note our own scientific publications.
- Singh, N., Singh, N., & Dhall, A. (2017). Continuous Multimodal Emotion Recognition Approach for AVEC 2017. arXiv preprint arXiv:1709.05861.
- Vielzeuf, V., Pateux, S., & Jurie, F. (2017, November). Temporal multimodal fusion for video emotion classification in the wild. In Proceedings of the 19th ACM International Conference on Multimodal Interaction (pp. 569-576). ACM.
- Tao, F., & Liu, G. (2018, April). Advanced LSTM: A study about better time dependency modeling in emotion recognition. In 2018 IEEE International Conference on Acoustics, Speech and Signal Processing (ICASSP) (pp. 2906-2910). IEEE.
- Tian, L., Muszynski, M., Lai, C., Moore, J. D., Kostoulas, T., Lombardo, P., … & Chanel, G. (2017, October). Recognizing induced emotions of movie audiences: Are induced and perceived emotions the same?. In Affective Computing and Intelligent Interaction (ACII), 2017 Seventh International Conference on (pp. 28-35). IEEE.
- Gamage, K. W., Sethu, V., & Ambikairajah, E. (2017, October). Modeling variable length phoneme sequences—A step towards linguistic information for speech emotion recognition in wider world. In Affective Computing and Intelligent Interaction (ACII), 2017 Seventh International Conference on (pp. 518-523). IEEE.
- Knyazev, B., Shvetsov, R., Efremova, N., & Kuharenko, A. (2017). Convolutional neural networks pretrained on large face recognition datasets for emotion classification from video. arXiv preprint arXiv:1711.04598.
- Gaus, Y. F. A., Meng, H., & Jan, A. (2017, June). Decoupling Temporal Dynamics for Naturalistic Affect Recognition in a Two-Stage Regression Framework. In Cybernetics (CYBCONF), 2017 3rd IEEE International Conference on (pp. 1-6). IEEE.
- Cambria, E., Hazarika, D., Poria, S., Hussain, A., & Subramaanyam, R. B. V. (2017). Benchmarking multimodal sentiment analysis. arXiv preprint arXiv:1707.09538.
- Torres, J. M. M., & Stepanov, E. A. (2017, August). Enhanced face/audio emotion recognition: video and instance level classification using ConvNets and restricted Boltzmann Machines. In Proceedings of the International Conference on Web Intelligence (pp. 939-946). ACM.
- Siegert, I., Lotz, A. F., Egorow, O., & Wendemuth, A. (2017, September). Improving Speech-Based Emotion Recognition by Using Psychoacoustic Modeling and Analysis-by-Synthesis. In International Conference on Speech and Computer (pp. 445- 455). Springer, Cham.
- Huang, C. W., & Narayanan, S. S. (2017, July). Deep convolutional recurrent neural network with attention mechanism for robust speech emotion recognition. In Multimedia and Expo (ICME), 2017 IEEE International Conference on (pp. 583- 588). IEEE.
- Dhall, A., Goecke, R., Joshi, J., Wagner, M., & Gedeon, T. (2013, December). Emotion recognition in the wild challenge 2013. In Proceedings of the 15th ACM on International conference on multimodal interaction (pp. 509-516). ACM.
- Dhall, A., Goecke, R., Joshi, J., Sikka, K., & Gedeon, T. (2014, November). Emotion recognition in the wild challenge 2014: Baseline, data and protocol. In Proceedings of the 16th International Conference on Multimodal Interaction (pp. 461-466). ACM.
- Liu, M., Wang, R., Li, S., Shan, S., Huang, Z., & Chen, X. (2014, November). Combining multiple kernel methods on riemannian manifold for emotion recognition in the wild. In Proceedings of the 16th International Conference on Multimodal Interaction (pp. 494-501). ACM.
- Dhall, A., Ramana Murthy, O. V., Goecke, R., Joshi, J., & Gedeon, T. (2015, November). Video and image based emotion recognition challenges in the wild: Emotiw 2015. In Proceedings of the 2015 ACM on International Conference on Multimodal Interaction (pp. 423-426). ACM.
- Savran, A., Cao, H., Shah, M., Nenkova, A., & Verma, R. (2012, October). Combining video, audio and lexical indicators of affect in spontaneous conversation via particle filtering. In Proceedings of the 14th ACM international conference on Multimodal interaction (pp. 485-492). ACM.
- Poria, S., Cambria, E., & Gelbukh, A. F. (2015, September). Deep Convolutional Neural Network Textual Features and Multiple Kernel Learning for Utterance-level Multimodal Sentiment Analysis. In EMNLP (pp. 2539-2544).
- Zheng, W., Xin, M., Wang, X., & Wang, B. (2014). A novel speech emotion recognition method via incomplete sparse least square regression. IEEE Signal Processing Letters, 21(5), 569-572.
- Bhattacharya, A., Wu, W., & Yang, Z. (2012). Quality of experience evaluation of voice communication: an affect-based approach. Human-centric Computing and Information Sciences, 2(1), 7.
- Bone, D., Lee, C. C., & Narayanan, S. (2014). Robust unsupervised arousal rating: A rule-based framework withknowledge-inspired vocal features. IEEE transactions on affective computing, 5(2), 201-213.
- Liu, M., Wang, R., Huang, Z., Shan, S., & Chen, X. (2013, December). Partial least squares regression on grassmannian manifold for emotion recognition. In Proceedings of the 15th ACM on International conference on multimodal interaction (pp. 525-530). ACM.
- Audhkhasi, K., & Narayanan, S. (2013). A globally-variant locally-constant model for fusion of labels from multiple diverse experts without using reference labels. IEEE transactions on pattern analysis and machine intelligence, 35(4), 769-783.
- Mariooryad, S., & Busso, C. (2013). Exploring cross-modality affective reactions for audiovisual emotion recognition. IEEE Transactions on affective computing, 4(2), 183-196.
- Chen, J., Chen, Z., Chi, Z., & Fu, H. (2014, November). Emotion recognition in the wild with feature fusion and multiple kernel learning. In Proceedings of the 16th International Conference on Multimodal Interaction (pp. 508-513). ACM.
- Rosenberg, A. (2012). Classifying Skewed Data: Importance Weighting to Optimize Average Recall. In Interspeech (pp. 2242-2245).
- Sun, R., & Moore, E. (2011). Investigating glottal parameters and teager energy operators in emotion recognition. Affective computing and intelligent interaction, 425-434.
- Sun, B., Li, L., Zuo, T., Chen, Y., Zhou, G., & Wu, X. (2014, November). Combining multimodal features with hierarchical classifier fusion for emotion recognition in the wild. In Proceedings of the 16th International Conference on Multimodal Interaction (pp. 481-486). ACM.
- Mariooryad, S., & Busso, C. (2015). Correcting time-continuous emotional labels by modeling the reaction lag of evaluators. IEEE Transactions on Affective Computing, 6(2), 97-108.
- Ivanov, A., & Riccardi, G. (2012, March). Kolmogorov-Smirnov test for feature selection in emotion recognition from speech. In Acoustics, Speech and Signal Processing (ICASSP), 2012 IEEE International Conference on (pp. 5125-5128). IEEE.
- Mariooryad, S., & Busso, C. (2013, September). Analysis and compensation of the reaction lag of evaluators in continuous emotional annotations. In Affective Computing and Intelligent Interaction (ACII), 2013 Humaine Association Conference on (pp. 85-90). IEEE.
- Alonso-Martín, F., Malfaz, M., Sequeira, J., Gorostiza, J. F., & Salichs, M. A. (2013). A multimodal emotion detection system during human–robot interaction. Sensors, 13(11), 15549-15581.
- Moore, J. D., Tian, L., & Lai, C. (2014, April). Word-level emotion recognition using high-level features. In International Conference on Intelligent Text Processing and Computational Linguistics (pp. 17-31). Springer Berlin Heidelberg.
- Cao, H., Verma, R., & Nenkova, A. (2015). Speaker-sensitive emotion recognition via ranking: Studies on acted and spontaneous speech. Computer speech & language, 29(1), 186-202.
- Mariooryad, S., & Busso, C. (2014). Compensating for speaker or lexical variabilities in speech for emotion recognition. Speech Communication, 57, 1-12.
- Wu, C. H., Lin, J. C., & Wei, W. L. (2014). Survey on audiovisual emotion recognition: databases, features, and data fusion strategies. APSIPA transactions on signal and information processing, 3, e12.
- Busso, C., Mariooryad, S., Metallinou, A., & Narayanan, S. (2013). Iterative feature normalization scheme for automatic emotion detection from speech. IEEE transactions on Affective computing, 4(4), 386-397.
- Galanis, D., Karabetsos, S., Koutsombogera, M., Papageorgiou, H., Esposito, A., & Riviello, M. T. (2013, December). Classification of emotional speech units in call centre interactions. In Cognitive Infocommunications (CogInfoCom), 2013 IEEE 4th International Conference on (pp. 403-406). IEEE.
- Sidorov, M., Brester, C., Minker, W., & Semenkin, E. (2014, May). Speech-Based Emotion Recognition: Feature Selection by Self-Adaptive Multi-Criteria Genetic Algorithm. In LREC (pp. 3481-3485).
- Oflazoglu, C., & Yildirim, S. (2013). Recognizing emotion from Turkish speech using acoustic features. EURASIP Journal on Audio, Speech, and Music Processing, 2013(1), 26.
- Kaya, H., & Salah, A. A. (2016). Combining modality-specific extreme learning machines for emotion recognition in the wild. Journal on Multimodal User Interfaces, 10(2), 139-149.
- Amer, M. R., Siddiquie, B., Richey, C., & Divakaran, A. (2014, May). Emotion detection in speech using deep networks. In Acoustics, Speech and Signal Processing (ICASSP), 2014 IEEE International Conference on (pp. 3724-3728). IEEE.
- Poria, S., Chaturvedi, I., Cambria, E., & Hussain, A. (2016, December). Convolutional MKL based multimodal emotion recognition and sentiment analysis. In Data Mining (ICDM), 2016 IEEE 16th International Conference on (pp. 439-448). IEEE.
- Kaya, H., Çilli, F., & Salah, A. A. (2014, November). Ensemble CCA for continuous emotion prediction. In Proceedings of the 4th International Workshop on Audio/Visual Emotion Challenge (pp. 19-26). ACM.
- Mariooryad, S., Lotfian, R., & Busso, C. (2014, September). Building a naturalistic emotional speech corpus by retrieving expressive behaviors from existing speech corpora. In INTERSPEECH (pp. 238-242).
- Busso, C., Parthasarathy, S., Burmania, A., AbdelWahab, M., Sadoughi, N., & Provost, E. M. (2017). MSP-IMPROV: An acted corpus of dyadic interactions to study emotion perception. IEEE Transactions on Affective Computing, 8(1), 67-80.
- Jin, Q., Li, C., Chen, S., & Wu, H. (2015, April). Speech emotion recognition with acoustic and lexical features. In Acoustics, Speech and Signal Processing (ICASSP), 2015 IEEE International Conference on (pp. 4749-4753). IEEE.
- Peng, S. O. N. G., Yun, J. I. N., Li, Z. H. A. O., & Minghai, X. I. N. (2014). Speech emotion recognition using transfer learning. IEICE TRANSACTIONS on Information and Systems, 97(9), 2530-2532.
- Huang, D. Y., Zhang, Z., & Ge, S. S. (2014). Speaker state classification based on fusion of asymmetric simple partial least squares (SIMPLS) and support vector machines. Computer Speech & Language, 28(2), 392-419.
- Sun, Y., Wen, G., & Wang, J. (2015). Weighted spectral features based on local Hu moments for speech emotion recognition. Biomedical signal processing and control, 18, 80-90.
- Kaya, H., Gürpinar, F., Afshar, S., & Salah, A. A. (2015, November). Contrasting and combining least squares based learners for emotion recognition in the wild. In Proceedings of the 2015 ACM on International Conference on Multimodal Interaction (pp. 459-466). ACM.
- Banda, N., & Robinson, P. (2011, November). Noise analysis in audio-visual emotion recognition. In Proceedings of the International Conference on Multimodal Interaction (pp. 1-4).
- Chen, S., & Jin, Q. (2015, October). Multi-modal dimensional emotion recognition using recurrent neural networks. In Proceedings of the 5th International Workshop on Audio/Visual Emotion Challenge (pp. 49-56). ACM.
- Audhkhasi, K., Sethy, A., Ramabhadran, B., & Narayanan, S. S. (2012, March). Creating ensemble of diverse maximum entropy models. In Acoustics, Speech and Signal Processing (ICASSP), 2012 IEEE International Conference on (pp. 4845-4848). IEEE.
- Lubis, N., Sakti, S., Neubig, G., Toda, T., Purwarianti, A., & Nakamura, S. (2016). Emotion and its triggers in human spoken dialogue: Recognition and analysis. In Situated Dialog in Speech-Based Human-Computer Interaction (pp. 103-110). Springer International Publishing.
- Song, P., Jin, Y., Zha, C., & Zhao, L. (2014). Speech emotion recognition method based on hidden factor analysis. Electronics Letters, 51(1), 112-114.
- Dhall, A., Goecke, R., Joshi, J., Wagner, M., & Gedeon, T. (2013, December). Emotion recognition in the wild challenge (EmotiW) challenge and workshop summary. In Proceedings of the 15th ACM on International conference on multimodal interaction (pp. 371-372). ACM.
- Chen, L., Yoon, S. Y., Leong, C. W., Martin, M., & Ma, M. (2014, November). An initial analysis of structured video interviews by using multimodal emotion detection. In Proceedings of the 2014 workshop on Emotion Representation and Modelling in Human-Computer-Interaction-Systems (pp. 1-6). ACM.
- Brester, C., Semenkin, E., Sidorov, M., & Minker, W. (2014). Self-adaptive multi-objective genetic algorithms for feature selection. In Proceedings of International Conference on Engineering and Applied Sciences Optimization (pp. 1838-1846).
- Tian, L., Lai, C., & Moore, J. (2015, April). Recognizing emotions in dialogues with disfluencies and non-verbal vocalisations. In Proceedings of the 4th Interdisciplinary Workshop on Laughter and Other Non-verbal Vocalisations in Speech (Vol. 14, p. 15).
- Lopez-Otero, P., Docio-Fernandez, L., & Garcia-Mateo, C. (2014). iVectors for continuous emotion recognition. Training, 45, 50.
- Bojanic, M., Crnojevic, V., & Delic, V. (2012, September). Application of neural networks in emotional speech recognition. In Neural Network Applications in Electrical Engineering (NEUREL), 2012 11th Symposium on (pp. 223-226). IEEE.
- Kim, J. C., & Clements, M. A. (2015). Multimodal affect classification at various temporal lengths. IEEE Transactions on Affective Computing, 6(4), 371-384.
- Bone, D., Lee, C. C., Potamianos, A., & Narayanan, S. S. (2014). An investigation of vocal arousal dynamics in child-psychologist interactions using synchrony measures and a conversation-based model. In INTERSPEECH (pp. 218-222).
- Day, M. (2013, December). Emotion recognition with boosted tree classifiers. In Proceedings of the 15th ACM on International conference on multimodal interaction (pp. 531-534). ACM.
- Sidorov, M., Ultes, S., & Schmitt, A. (2014, May). Comparison of Gender-and Speaker-adaptive Emotion Recognition. In LREC (pp. 3476-3480).
- Tian, L., Moore, J. D., & Lai, C. (2015, September). Emotion recognition in spontaneous and acted dialogues. In Affective Computing and Intelligent Interaction (ACII), 2015 International Conference on (pp. 698-704). IEEE.
- Sun, B., Li, L., Zhou, G., Wu, X., He, J., Yu, L., … & Wei, Q. (2015, November). Combining multimodal features within a fusion network for emotion recognition in the wild. In Proceedings of the 2015 ACM on International Conference on Multimodal Interaction (pp. 497-502). ACM.
- Ellis, J. G., Lin, W. S., Lin, C. Y., & Chang, S. F. (2014, December). Predicting evoked emotions in video. In Multimedia (ISM), 2014 IEEE International Symposium on (pp. 287-294). IEEE.
- Brester, C., Semenkin, E., Kovalev, I., Zelenkov, P., & Sidorov, M. (2015, May). Evolutionary feature selection for emotion recognition in multilingual speech analysis. In Evolutionary Computation (CEC), 2015 IEEE Congress on (pp. 2406-2411). IEEE.
- Zhang, B., Provost, E. M., Swedberg, R., & Essl, G. (2015, January). Predicting Emotion Perception Across Domains: A Study of Singing and Speaking. In AAAI (pp. 1328-1335).
- Brester, C., Sidorov, M., & Semenkin, E. (2014). Speech-based emotion recognition: Application of collective decision making concepts. In Proceedings of the 2nd International Conference on Computer Science and Artificial Intelligence (ICCSAI2014) (pp. 216-220).
- Cao, H., Savran, A., Verma, R., & Nenkova, A. (2015). Acoustic and lexical representations for affect prediction in spontaneous conversations. Computer speech & language, 29(1), 203-217.
- Sidorov, M., Brester, C., Semenkin, E., & Minker, W. (2014, September). Speaker state recognition with neural network-based classification and self-adaptive heuristic feature selection. In Informatics in Control, Automation and Robotics (ICINCO), 2014 11th International Conference on (Vol. 1, pp. 699-703). IEEE.
- Tickle, A., Raghu, S., & Elshaw, M. (2013). Emotional recognition from the speech signal for a virtual education agent. In Journal of Physics: Conference Series (Vol. 450, No. 1, p. 012053). IOP Publishing.
- Vinciarelli, A., & Mohammadi, G. (2014). A survey of personality computing. IEEE Transactions on Affective Computing, 5(3), 273-291.
- Pohjalainen, J., Räsänen, O., & Kadioglu, S. (2015). Feature selection methods and their combinations in high-dimensional classification of speaker likability, intelligibility and personality traits. Computer Speech & Language, 29(1), 145-171.
- Ivanov, A. V., Riccardi, G., Sporka, A. J., & Franc, J. (2011). Recognition of Personality Traits from Human Spoken Conversations. In INTERSPEECH (pp. 1549-1552).
- Chastagnol, C., & Devillers, L. (2012). Personality traits detection using a parallelized modified SFFS algorithm. computing, 15, 16.
- Alam, F., & Riccardi, G. (2013, August). Comparative study of speaker personality traits recognition in conversational and broadcast news speech. In INTERSPEECH (pp. 2851-2855).
- Alam, F., & Riccardi, G. (2014, May). Fusion of acoustic, linguistic and psycholinguistic features for speaker personality traits recognition. In Acoustics, Speech and Signal Processing (ICASSP), 2014 IEEE International Conference on (pp. 955-959). IEEE.
- Wagner, J., Lingenfelser, F., & André, E. (2012). A Frame Pruning Approach for Paralinguistic Recognition Tasks. In INTERSPEECH (pp. 274-277).
- Feese, S., Muaremi, A., Arnrich, B., Troster, G., Meyer, B., & Jonas, K. (2011, October). Discriminating individually considerate and authoritarian leaders by speech activity cues. In Privacy, Security, Risk and Trust (PASSAT) and 2011 IEEE Third Inernational Conference on Social Computing (SocialCom), 2011 IEEE Third International Conference on (pp. 1460-1465). IEEE.
- Liu, G., & Hansen, J. H. (2014). Supra-segmental feature based speaker trait detection. In Proc. Odyssey.
- Liu, C. J., Wu, C. H., & Chiu, Y. H. (2013, October). BFI-based speaker personality perception using acoustic-prosodic features. In Signal and Information Processing Association Annual Summit and Conference (APSIPA), 2013 Asia-Pacific (pp. 1-6). IEEE.
- Grünerbl, A., Muaremi, A., Osmani, V., Bahle, G., Oehler, S., Tröster, G., … & Lukowicz, P. (2015). Smartphone-based recognition of states and state changes in bipolar disorder patients. IEEE Journal of Biomedical and Health Informatics, 19(1), 140-148.
- Gravenhorst, F., Muaremi, A., Bardram, J., Grünerbl, A., Mayora, O., Wurzer, G., … & Tröster, G. (2015). Mobile phones as medical devices in mental disorder treatment: an overview. Personal and Ubiquitous Computing, 19(2), 335-353.
- Cummins, N., Joshi, J., Dhall, A., Sethu, V., Goecke, R., & Epps, J. (2013, October). Diagnosis of depression by behavioural signals: a multimodal approach. In Proceedings of the 3rd ACM international workshop on Audio/visual emotion challenge (pp. 11-20). ACM.
- Alghowinem, S., Goecke, R., Wagner, M., Epps, J., Breakspear, M., & Parker, G. (2012, May). From Joyous to Clinically Depressed: Mood Detection Using Spontaneous Speech. In FLAIRS Conference.
- Joshi, J., Goecke, R., Alghowinem, S., Dhall, A., Wagner, M., Epps, J., … & Breakspear, M. (2013). Multimodal assistive technologies for depression diagnosis and monitoring. Journal on Multimodal User Interfaces, 7(3), 217-228.
- Alghowinem, S., Goecke, R., Wagner, M., Epps, J., Breakspear, M., & Parker, G. (2013, May). Detecting depression: a comparison between spontaneous and read speech. In Acoustics, Speech and Signal Processing (ICASSP), 2013 IEEE International Conference on (pp. 7547-7551). IEEE.
- Cummins, N., Epps, J., Sethu, V., Breakspear, M., & Goecke, R. (2013, August). Modeling spectral variability for the classification of depressed speech. In Interspeech (pp. 857-861).
- Alghowinem, S., Goecke, R., Wagner, M., Epps, J., Gedeon, T., Breakspear, M., & Parker, G. (2013, May). A comparative study of different classifiers for detecting depression from spontaneous speech. In Acoustics, Speech and Signal Processing (ICASSP), 2013 IEEE International Conference on (pp. 8022-8026). IEEE.
- Gupta, R., Malandrakis, N., Xiao, B., Guha, T., Van Segbroeck, M., Black, M., … & Narayanan, S. (2014, November). Multimodal prediction of affective dimensions and depression in human-computer interactions. In Proceedings of the 4th International Workshop on Audio/Visual Emotion Challenge (pp. 33-40). ACM.
- Karam, Z. N., Provost, E. M., Singh, S., Montgomery, J., Archer, C., Harrington, G., & Mcinnis, M. G. (2014, May). Ecologically valid long-term mood monitoring of individuals with bipolar disorder using speech. In Acoustics, Speech and Signal Processing (ICASSP), 2014 IEEE International Conference on (pp. 4858-4862). IEEE.
- Mitra, V., Shriberg, E., McLaren, M., Kathol, A., Richey, C., Vergyri, D., & Graciarena, M. (2014, November). The SRI AVEC-2014 evaluation system. In Proceedings of the 4th International Workshop on Audio/Visual Emotion Challenge (pp. 93-101). ACM.
- Sidorov, M., & Minker, W. (2014, November). Emotion recognition and depression diagnosis by acoustic and visual features: A multimodal approach. In Proceedings of the 4th International Workshop on Audio/Visual Emotion Challenge (pp. 81-86). ACM.
- Kaya, H., & Salah, A. A. (2014, November). Eyes whisper depression: A cca based multimodal approach. In Proceedings of the 22nd ACM international conference on Multimedia (pp. 961-964). ACM.
- Hönig, F., Batliner, A., Nöth, E., Schnieder, S., & Krajewski, J. (2014, September). Automatic modelling of depressed speech: relevant features and relevance of gender. In INTERSPEECH (pp. 1248-1252).
- Alghowinem, S., Goecke, R., Wagner, M., Epps, J., Parker, G., & Breakspear, M. (2013). Characterising depressed speech for classification. In Interspeech (pp. 2534-2538).
- Asgari, M., Shafran, I., & Sheeber, L. B. (2014, September). Inferring clinical depression from speech and spoken utterances. In Machine Learning for Signal Processing (MLSP), 2014 IEEE International Workshop on (pp. 1-5). IEEE.
- Lopez-Otero, P., Docio-Fernandez, L., & Garcia-Mateo, C. (2014, May). A study of acoustic features for the classification of depressed speech. In Information and Communication Technology, Electronics and Microelectronics (MIPRO), 2014 37th International Convention on (pp. 1331-1335). IEEE.
- Lopez-Otero, P., Dacia-Fernandez, L., & Garcia-Mateo, C. (2014, March). A study of acoustic features for depression detection. In Biometrics and Forensics (IWBF), 2014 International Workshop on (pp. 1-6). IEEE.
- Nasir, M., Baucom, B. R., Georgiou, P., & Narayanan, S. (2017). Predicting couple therapy outcomes based on speech acoustic features. PloS one, 12(9), e0185123.
- Rao, H., Clements, M. A., Li, Y., Swanson, M. R., Piven, J., & Messinger, D. S. (2017). Paralinguistic Analysis of Children’s Speech in Natural Environments. In Mobile Health (pp. 219-238). Springer, Cham.
- Chowdhury, S. A. (2017). Computational modeling of turn-taking dynamics in spoken conversations (Doctoral dissertation, University of Trento).
- Silber-Varod, V., Lerner, A., & Jokisch, O. (2017). Automatic Speaker’s Role Classification with a Bottom-up Acoustic Feature Selection. In Proc. GLU 2017 International Workshop on Grounding Language Understanding (pp. 52-56).
- Rehg, J., Abowd, G., Rozga, A., Romero, M., Clements, M., Sclaroff, S., … & Rao, H. (2013). Decoding children’s social behavior. In Proceedings of the IEEE conference on computer vision and pattern recognition (pp. 3414-3421).
- Wagner, J., Lingenfelser, F., Baur, T., Damian, I., Kistler, F., & André, E. (2013, October). The social signal interpretation (SSI) framework: multimodal signal processing and recognition in real-time. In Proceedings of the 21st ACM international conference on Multimedia (pp. 831-834). ACM.
- Black, M. P., Katsamanis, A., Baucom, B. R., Lee, C. C., Lammert, A. C., Christensen, A., … & Narayanan, S. S. (2013). Toward automating a human behavioral coding system for married couples’ interactions using speech acoustic features. Speech Communication, 55(1), 1-21.
- Lee, C. C., Katsamanis, A., Black, M. P., Baucom, B. R., Christensen, A., Georgiou, P. G., & Narayanan, S. S. (2014). Computing vocal entrainment: A signal-derived PCA-based quantification scheme with application to affect analysis in married couple interactions. Computer Speech & Language, 28(2), 518-539.
- Black, M., Georgiou, P. G., Katsamanis, A., Baucom, B. R., & Narayanan, S. S. (2011, August). ” You made me do it”: Classification of Blame in Married Couples’ Interactions by Fusing Automatically Derived Speech and Language Information. In Interspeech (pp. 89-92).
- Lubold, N., & Pon-Barry, H. (2014, November). Acoustic-prosodic entrainment and rapport in collaborative learning dialogues. In Proceedings of the 2014 ACM workshop on Multimodal Learning Analytics Workshop and Grand Challenge (pp. 5-12). ACM.
- Neiberg, D., & Gustafson, J. (2011). Predicting Speaker Changes and Listener Responses with and without Eye-Contact. In INTERSPEECH (pp. 1565-1568).
- Wagner, J., Lingenfelser, F., & André, E. (2013). Using phonetic patterns for detecting social cues in natural conversations. In INTERSPEECH (pp. 168-172).
- Avril, M., Leclère, C., Viaux, S., Michelet, S., Achard, C., Missonnier, S., … & Chetouani, M. (2014). Social signal processing for studying parent–infant interaction. Frontiers in psychology, 5, 1437.
- Jones, H. E., Sabouret, N., Damian, I., Baur, T., André, E., Porayska-Pomsta, K., & Rizzo, P. (2014). Interpreting social cues to generate credible affective reactions of virtual job interviewers. arXiv preprint arXiv:1402.5039.
- Zhao, R., Sinha, T., Black, A. W., & Cassell, J. (2016, September). Automatic recognition of conversational strategies in the service of a socially-aware dialog system. In 17th Annual Meeting of the Special Interest Group on Discourse and Dialogue (p. 381).
- Rasheed, U., Tahir, Y., Dauwels, S., Dauwels, J., Thalmann, D., & Magnenat-Thalmann, N. (2013, October). Real-Time Comprehensive Sociometrics for Two-Person Dialogs. In HBU (pp. 196-208).
- Sapru, A., & Bourlard, H. (2015). Automatic recognition of emergent social roles in small group interactions. IEEE Transactions on Multimedia, 17(5), 746-760.
- Muaremi, A., Arnrich, B., & Tröster, G. (2013). Towards measuring stress with smartphones and wearable devices during workday and sleep. BioNanoScience, 3(2), 172-183.
- Van Segbroeck, M., Travadi, R., Vaz, C., Kim, J., Black, M. P., Potamianos, A., & Narayanan, S. S. (2014, September). Classification of cognitive load from speech using an i-vector framework. In INTERSPEECH (pp. 751-755).
- Aguiar, A. C., Kaiseler, M., Meinedo, H., Abrudan, T. E., & Almeida, P. R. (2013, September). Speech stress assessment using physiological and psychological measures. In Proceedings of the 2013 ACM conference on Pervasive and ubiquitous computing adjunct publication (pp. 921-930). ACM.
- Li, M. (2014). Automatic recognition of speaker physical load using posterior probability based features from acoustic and phonetic tokens.
- Niewiadomski, R., Hofmann, J., Urbain, J., Platt, T., Wagner, J., Piot, B., … & Geist, M. (2013, May). Laugh-aware virtual agent and its impact on user amusement. In Proceedings of the 2013 international conference on Autonomous agents
- Gupta, R., Audhkhasi, K., Lee, S., & Narayanan, S. (2013). Paralinguistic event detection from speech using probabilistic time-series smoothing and masking. In Interspeech (pp. 173-177).
- Oh, J., Cho, E., & Slaney, M. (2013, August). Characteristic contours of syllabic-level units in laughter. In Interspeech (pp. 158-162).
- Pohjalainen, J., Räsänen, O., & Kadioglu, S. (2015). Feature selection methods and their combinations in high-dimensional classification of speaker likability, intelligibility and personality traits. Computer Speech & Language, 29(1), 145-171.
- Carlson, N. A. (2017, September). Simple Acoustic-Prosodic Models of Confidence and Likability are Associated with Long-Term Funding Outcomes for Entrepreneurs. In International Conference on Social Informatics (pp. 3-16). Springer, Cham.
- Bone, D., Lee, C. C., Black, M. P., Williams, M. E., Lee, S., Levitt, P., & Narayanan, S. (2014). The psychologist as an interlocutor in autism spectrum disorder assessment: Insights from a study of spontaneous prosody. Journal of Speech, Language, and Hearing Research, 57(4), 1162-1177.
- Räsänen, O., & Pohjalainen, J. (2013, August). Random subset feature selection in automatic recognition of developmental disorders, affective states, and level of conflict from speech. In INTERSPEECH (pp. 210-214).
- Bone, D., Chaspari, T., Audhkhasi, K., Gibson, J., Tsiartas, A., Van Segbroeck, M., … & Narayanan, S. (2013). Classifying language-related developmental disorders from speech cues: the promise and the potential confounds. In INTERSPEECH (pp. 182-186).
- Reidsma, D., de Kok, I., Neiberg, D., Pammi, S. C., van Straalen, B., Truong, K., & van Welbergen, H. (2011). Continuous interaction with a virtual human. Journal on Multimodal User Interfaces, 4(2), 97-118.
- Bevacqua, E., De Sevin, E., Hyniewska, S. J., & Pelachaud, C. (2012). A listener model: introducing personality traits. Journal on Multimodal User Interfaces, 6(1-2), 27-38.
- Kopp, S., van Welbergen, H., Yaghoubzadeh, R., & Buschmeier, H. (2014). An architecture for fluid real-time conversational agents: integrating incremental output generation and input processing. Journal on Multimodal User Interfaces, 8(1), 97-108.
- Neiberg, D., & Truong, K. P. (2011, May). Online detection of vocal listener responses with maximum latency constraints. In Acoustics, Speech and Signal Processing (ICASSP), 2011 IEEE International Conference on (pp. 5836-5839). IEEE.
- Maat, M. (2011). Response selection and turn-taking for a sensitive artificial listening agent. University of Twente.
- Gebhard, P., Baur, T., Damian, I., Mehlmann, G., Wagner, J., & André, E. (2014, May). Exploring interaction strategies for virtual characters to induce stress in simulated job interviews. In Proceedings of the 2014 international conference on Autonomous agents and multi-agent systems (pp. 661-668). International Foundation for Autonomous Agents and Multiagent Systems.
- Potamitis, I., Ntalampiras, S., Jahn, O., & Riede, K. (2014). Automatic bird sound detection in long real-field recordings: Applications and tools. Applied Acoustics, 80, 1-9.
- Goëau, H., Glotin, H., Vellinga, W. P., Planqué, R., Rauber, A., & Joly, A. (2014, September). LifeCLEF bird identification task 2014. In CLEF2014.
- Lasseck, M. (2014). Large-scale Identification of Birds in Audio Recordings. In CLEF (Working Notes) (pp. 643-653).
- Lasseck, M. (2015, September). Towards automatic large-scale identification of birds in audio recordings. In International Conference of the Cross-Language Evaluation Forum for European Languages (pp. 364-375). Springer International Publishing.
Lukacs, G., Jani, M., & Takacs, G. (2013, September). Acoustic feature mining for mixed speech and music playlist generation. In ELMAR, 2013 55th International Symposium (pp. 275-278). IEEE.
- Black, A. W., Bunnell, H. T., Dou, Y., Muthukumar, P. K., Metze, F., Perry, D., … & Vaughn, C. (2012, March). Articulatory features for expressive speech synthesis. In Acoustics, Speech and Signal Processing (ICASSP), 2012 IEEE International Conference on (pp. 4005-4008). IEEE.
- Steidl, S., Polzehl, T., Bunnell, H. T., Dou, Y., Muthukumar, P. K., Perry, D., … & Metze, F. (2012). Emotion identification for evaluation of synthesized emotional speech.
- Gallardo-Antolín, A., Montero, J. M., & King, S. (2014). A comparison of open-source segmentation architectures for dealing with imperfect data from the media in speech synthesis.
- Alhanai, T., Au, R., & Glass, J. (2017, December). Spoken language biomarkers for detecting cognitive impairment. In Automatic Speech Recognition and Understanding Workshop (ASRU), 2017 IEEE (pp. 409-416). IEEE.
- Bayestehtashk, A., Asgari, M., Shafran, I., & McNames, J. (2015). Fully automated assessment of the severity of Parkinson’s disease from speech. Computer speech & language, 29(1), 172-185.
- Bocklet, T., Steidl, S., Nöth, E., & Skodda, S. (2013). Automatic evaluation of parkinson’s speech-acoustic, prosodic and voice related cues. In Interspeech (pp. 1149-1153).
- Orozco-Arroyave, J. R., Hönig, F., Arias-Londoño, J. D., Vargas-Bonilla, J. F., Daqrouq, K., Skodda, S., … & Nöth, E. (2016). Automatic detection of Parkinson’s disease in running speech spoken in three different languages. The Journal of the Acoustical Society of America, 139(1), 481-500.
- Kim, J., Nasir, M., Gupta, R., Van Segbroeck, M., Bone, D., Black, M. P., … & Narayanan, S. S. (2015, September). Automatic estimation of parkinson’s disease severity from diverse speech tasks. In INTERSPEECH (pp. 914-918).
- Pompili, A., Abad, A., Romano, P., Martins, I. P., Cardoso, R., Santos, H., … & Ferreira, J. J. (2017, August). Automatic Detection of Parkinson’s Disease: An Experimental Analysis of Common Speech Production Tasks Used for Diagnosis.
In International Conference on Text, Speech, and Dialogue (pp. 411-419). Springer, Cham.
- Gajšek, R., Mihelic, F., & Dobrišek, S. (2013). Speaker state recognition using an HMM-based feature extraction method. Computer Speech & Language, 27(1), 135-150.
- Bone, D., Li, M., Black, M. P., & Narayanan, S. S. (2014). Intoxicated speech detection: A fusion framework with speaker-normalized hierarchical functionals and GMM supervectors. Computer speech & language, 28(2), 375-391.
- Suendermann-Oeft, D., Ramanarayanan, V., Teckenbrock, M., Neutatz, F., & Schmidt, D. (2015). HALEF: An Open-Source Standard-Compliant Telephony-Based Modular Spoken Dialog System: A Review and An Outlook. In Natural Language Dialog Systems and Intelligent Assistants (pp. 53-61). Springer International Publishing.
- Huang, C. L., Tsao, Y., Hori, C., & Kashioka, H. (2011, October). Feature normalization and selection for robust speaker state recognition. In Speech Database and Assessments (Oriental COCOSDA), 2011 International Conference on (pp. 102-105). IEEE.
Kim, J., Kumar, N., Tsiartas, A., Li, M., & Narayanan, S. S. (2015). Automatic intelligibility classification of sentence-level pathological speech. Computer speech & language, 29(1), 132-144.
- Lefter, I., Rothkrantz, L. J., & Burghouts, G. J. (2013). A comparative study on automatic audio–visual fusion for aggression detection using meta-information. Pattern Recognition Letters, 34(15), 1953-1963.
- Gosztolya, G., & Tóth, L. (2017). DNN-Based Feature Extraction for Conflict Intensity Estimation From Speech. IEEE Signal Processing Letters, 24(12), 1837-1841.
Audhkhasi, K., Zavou, A. M., Georgiou, P. G., & Narayanan, S. S. (2014). Theoretical analysis of diversity in an ensemble of automatic speech recognition systems. IEEE/ACM Transactions on Audio, Speech, and Language Processing, 22(3), 711-726.
- Forbes-Riley, K., Litman, D., Friedberg, H., & Drummond, J. (2012, June). Intrinsic and extrinsic evaluation of an automatic user disengagement detector for an uncertainty-adaptive spoken dialogue system. In Proceedings of the 2012 Conference of the North American Chapter of the Association for Computational Linguistics: Human Language Technologies (pp. 91-102). Association for Computational Linguistics
- Litman, D. J., Friedberg, H., & Forbes-Riley, K. (2012). Prosodic Cues to Disengagement and Uncertainty in Physics Tutorial Dialogues. In INTERSPEECH (pp. 755-758).
- Cmejla, R., Rusz, J., Bergl, P., & Vokral, J. (2013). Bayesian changepoint detection for the automatic assessment of fluency and articulatory disorders. Speech Communication, 55(1), 178-189.
- Chalasani, T. (2017). AUTOMATED ASSESSMENT FOR THE THERAPY SUCCESS OF FOREIGN ACCENT SYNDROME: Based on Emotional Temperature.
Kalantarian, H., & Sarrafzadeh, M. (2015). Audio-based detection and evaluation of eating behavior using the smartwatch platform. Computers in biology and medicine, 65, 1-9.
- Metze, F., Rawat, S., & Wang, Y. (2014, July). Improved audio features for large-scale multimedia event detection. In Multimedia and Expo (ICME), 2014 IEEE International Conference on (pp. 1-6). IEEE.
- Rawat, S., Schulam, P. F., Burger, S., Ding, D., Wang, Y., & Metze, F. (2013). Robust audio-codebooks for large-scale event detection in consumer videos.
- Avila, S., Moreira, D., Perez, M., Moraes, D., Cota, I., Testoni, V., … & Rocha, A. (2014). RECOD at MediaEval 2014: Violent scenes detection task. In CEUR Workshop Proceedings. CEUR-WS.
Tran, T., Mariooryad, S., & Busso, C. (2013, May). Audiovisual corpus to analyze whisper speech. In Acoustics, Speech and Signal Processing (ICASSP), 2013 IEEE International Conference on (pp. 8101-8105). IEEE.
- Mariooryad, S., Kannan, A., Hakkani-Tur, D., & Shriberg, E. (2014, May). Automatic characterization of speaking styles in educational videos. In Acoustics, Speech and Signal Processing (ICASSP), 2014 IEEE International Conference on (pp. 4848-4852). IEEE.
- Verkhodanova, V., Shapranov, V., & Kipyatkova, I. (2017, September). Hesitations in Spontaneous Speech: Acoustic Analysis and Detection. In International Conference on Speech and Computer (pp. 398-406). Springer, Cham.
- Lee, M., Kim, J., Truong, K., de Kort, Y., Beute, F., & IJsselsteijn, W. (2017, October). Exploring moral conflicts in speech: Multidisciplinary analysis of affect and stress. In Affective Computing and Intelligent Interaction (ACII), 2017 Seventh International Conference on (pp. 407-414). IEEE.
Ben Youssef, A., Shimodaira, H., & Braude, D. A. (2013). Articulatory features for speech-driven head motion synthesis. Proceedings of Interspeech, Lyon, France.
Fan, Y., & Xu, M. (2014, October). MediaEval 2014: THU-HCSIL Approach to Emotion in Music Task using Multi-level Regression. In MediaEval.
Heckmann, M. (2014, September). Steps towards more natural human-machine interaction via audio-visual word prominence detection. In International Workshop on Multimodal Analyses Enabling Artificial Agents in Human-Machine Interaction (pp. 15-24). Springer International Publishing.
- Hönig, F., Bocklet, T., Riedhammer, K., Batliner, A., & Nöth, E. (2012). The Automatic Assessment of Non-native Prosody: Combining Classical Prosodic Analysis with Acoustic Modelling. In INTERSPEECH (pp. 823-826).
- Finkelstein, S., Ogan, A., Vaughn, C., & Cassell, J. (2013). Alex: A virtual peer that identifies student dialect. In Proc. Workshop on Culturally-aware Technology Enhanced Learning in conjuction with EC-TEL 2013, Paphos, Cyprus, September 17.
- Weng, S., Chen, S., Yu, L., Wu, X., Cai, W., Liu, Z., … & Li, M. (2015, December). The SYSU system for the interspeech 2015 automatic speaker verification spoofing and countermeasures challenge. In Signal and Information Processing Association Annual Summit and Conference (APSIPA), 2015 Asia-Pacific (pp. 152-155). IEEE.
- Parthasarathy, S., & Busso, C. (2017, October). Predicting speaker recognition reliability by considering emotional content. In Affective Computing and Intelligent Interaction (ACII), 2017 Seventh International Conference on (pp. 434 – 439). IEEE.
- Lehner, B., Widmer, G., & Bock, S. (2015, August). A low-latency, real-time-capable singing voice detection method with LSTM recurrent neural networks. In Signal Processing Conference (EUSIPCO), 2015 23rd European (pp. 21-25). IEEE.
- Sha, C. Y., Yang, Y. H., Lin, Y. C., & Chen, H. H. (2013, May). Singing voice timbre classification of chinese popular music. In Acoustics, Speech and Signal Processing (ICASSP), 2013 IEEE International Conference on (pp. 734-738). IEEE.
Ghosh, A., & Riccardi, G. (2014, November). Recognizing human activities from smartphone sensor signals. In Proceedings of the 22nd ACM international conference on Multimedia (pp. 865-868). ACM.
Your Publication
is Missing?
You have published a scientific article, thesis, paper or similar with reference to audEERING and would like to be listed here? Then contact us info@audeering.com